The Hidden Linearity: Why AI's Foundations Go Beyond Neural Networks
- Alper KARAGÖL
- Jan 23, 2024
- 2 min read
The rise of artificial intelligence has ignited imaginations and fueled anxieties. Deep learning algorithms, with their complex neuronal landscapes, seem to dominate the narrative. But a lesser-known truth is revealed beneath the surface: a substantial portion of modern AI relies on a much simpler tool - linear regression. With roots stretching back to the 18th century, it has quietly become the hidden hero of the AI revolution.
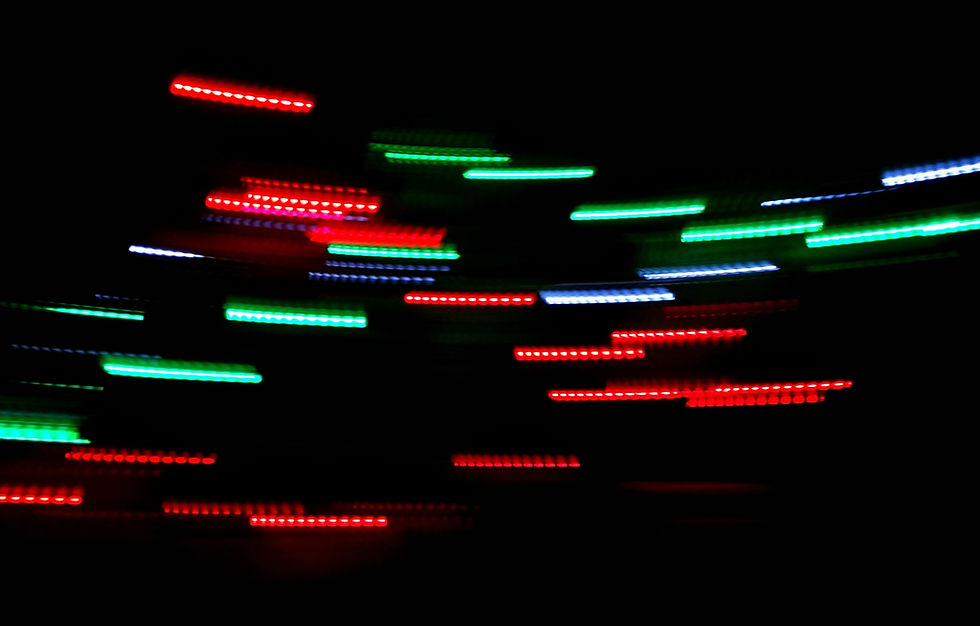
While neural networks are often in the spotlight, let's rewind the clock: Linear regression, developed in the 18th century by Adrien Marie Legendre, has a pedigree longer than any silicon chip. Initially employed in astronomy and cartography, linear regression found its niche in predicting trends and identifying correlations. Linear regression became a strong tool for statisticians and economists, unraveling economic patterns and forecasting market fluctuations!
But the true magic of linear regression lies not just in its historical journey, but in its enduring relevance. In the age of deep learning, where neural networks dominate the headlines, linear regression remains a cornerstone of AI. Its strengths shine in a world often obscured by complex algorithms. Unlike the opaque inner workings of neural networks, linear regression offers clarity. This clarity is invaluable for decision-making in crucial domains like healthcare and finance.
Secondly, its computational efficiency contributes the success. Training a complex neural network can be a daunting task, demanding vast amounts of data and processing power. Linear regression, on the other hand, scales gracefully with data size and excels at tasks with well-defined linear relationships. This makes it a go-to solution for resource-constrained scenarios or quick insights from smaller datasets.
Furthermore, linear regression acts as a building block for more intricate AI models. Many neural networks, despite their non-linearity, internally utilize linear algebra and optimization techniques rooted in linear regression. Understanding linear regression empowers us to grasp the fundamental mechanisms at play. This not only saves computational resources but also focuses holistic models on the truly meaningful signals within the data, preventing them from getting bogged down in irrelevant noise.
But like any tool, linear regression has limitations. It stumbles when dealing with complex, non-linear relationships. The human brain, for instance, is far more intricate than a linear model can capture.
Therefore, the story of AI is not a tale of one protagonist, but of a grand collaboration. Linear regression, with its interpretability, efficiency, and grounding in reality, can act as a compass, guiding holistic models toward navigating the complexities of the real world. By embracing this collaborative spirit, we can unlock the full potential of AI, not just for crunching numbers, but for truly understanding and shaping the world around us. While deep learning pushes the boundaries of complexity, it does so upon a foundation laid by centuries of mathematical ingenuity.
Comments